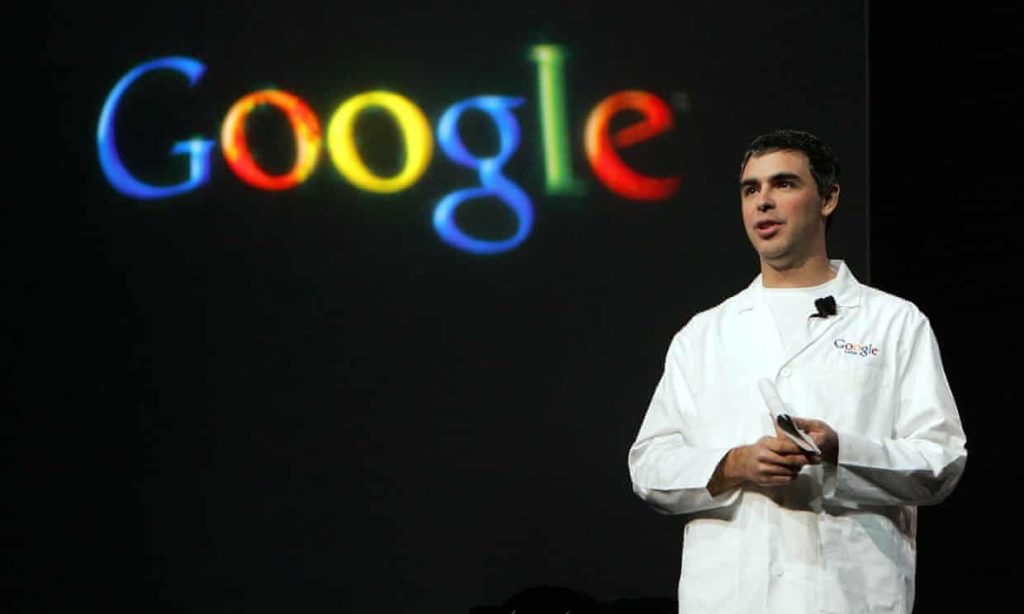
In 1998, a computer science PhD student called Larry Page submitted a patent for internet search based on an obscure piece of mathematics. The method, known today as PageRank, allowed the most relevant webpages to be found much more rapidly and accurately than ever before. The patent, initially owned by Stanford, was sold in 2005 for shares that are today worth more than $1bn. Page’s company, Google, has a net worth of well over $1tr.
It wasn’t Page, or Google’s cofounder Sergey Brin, who created the mathematics described in the patent. The equation they used is at least 100 years old, building on properties of matrices (mathematical structures akin to a spreadsheet of numbers). Similar methods were used by Chinese mathematicians more than two millennia ago. Page and Brin’s insight was to realise that by calculating what is known as the stationary distribution of a matrix describing connections on the world wide web, they could find the most popular sites more rapidly.
Applying the correct equation can suddenly solve an important practical problem, and completely change the world we live in.
The PageRank story is neither the first nor the most recent example of a little-known piece of mathematics transforming tech. In 2015, three engineers used the idea of gradient descent, dating back to the French mathematician Augustin-Louis Cauchy in the mid-19th century, to increase the time viewers spent watching YouTube by 2,000%. Their equation transformed the service from a place we went to for a few funny clips to a major consumer of our viewing time.
From the 1990s onwards, the financial industry has been built on variations of the diffusion equation, attributed to a variety of mathematicians including Einstein. Professional gamblers make use of logistic regression, developed by the Oxford statistician Sir David Cox in the 50s, to ensure they win at the expense of those punters who are less maths-savvy.
There is good reason to expect that there are more billion-dollar equations out there: generations-old mathematical theorems with the potential for new applications. The question is where to look for the next one.
A few candidates can be found in mathematical work in the latter part of the 20th century. One comes in the form of fractals, patterns that are self-similar, repeating on many different levels, like the branches of a tree or the shape of a broccoli head. Mathematicians developed a comprehensive theory of fractals in the 80s, and there was some excitement about applications that could store data more efficiently. Interest died out until recently, when a small community of computer scientists started showing how mathematical fractals can produce the most amazing, weird and wonderful patterns.
Another field of mathematics still looking for a money-making application is chaos theory, the best-known example of which is the butterfly effect: if a butterfly flaps its wings in the Amazon, we need to know about it in order to predict a storm in the North Atlantic. More generally, the theory tells us that, in order to accurately predict storms (or political events), we need to know about every tiny air disturbance on the entire planet. An impossible task. But chaos theory also points towards repeatable patterns. The Lorenz attractor is a model of the weather that, despite being chaotic, does produce somewhat regular and recognisable patterns. Given the uncertainty of the times we live in, it may be time to revive these ideas.
Obscure, generations-old theorems have been transformative in tech, and there are still plenty out there to be used
Some of my own research has focused on self-propelled particle models, which describe movements similar to those of bird flocks and fish schools. I now apply these models to better coordinate tactical formations in football and to scout players who move in ways that create more space for themselves and their teammates.Advertisement
Another related model is current reinforced random walks, which capture how ants build trails, and the structure of slime mould transportation networks. This model could take us from today’s computers – which have central processing units (CPUs) that make computations and separate memory chips to store information – to new forms of computation in which computation and memory are part of the same process. Like ant trails and slime mould, these new computers would benefit from being decentralised. Difficult computational problems, in particular in AI and computer vision, could be broken down in to smaller sub-problems and solved more rapidly.
Whenever there is a breakthrough application of an equation, we see a whole range of copycat imitations. The current boom in artificial intelligence is primarily driven by just two equations – gradient descent and logistic regression – put together to create what is known as a neural network. But history shows that the next big leap forward doesn’t come from repeatedly using the same mathematical trick. It comes instead from a completely new idea, read from the more obscure pages of the book of mathematics.
The challenge of finding the next billion-dollar equation is not simply one of knowing every page of that book. Page spotted the right problem to solve at the right time, and he persuaded the more theoretically inclined Brin to help him find the maths to help them. You don’t need to be a mathematical genius yourself in order to put the subject to good use. You just need to have a feeling for what equations are, and what they can and can’t do.
Mathematics still holds many hidden intellectual and financial riches. It is up to all of us to try to find them. The search for the next billion-dollar equation is on.
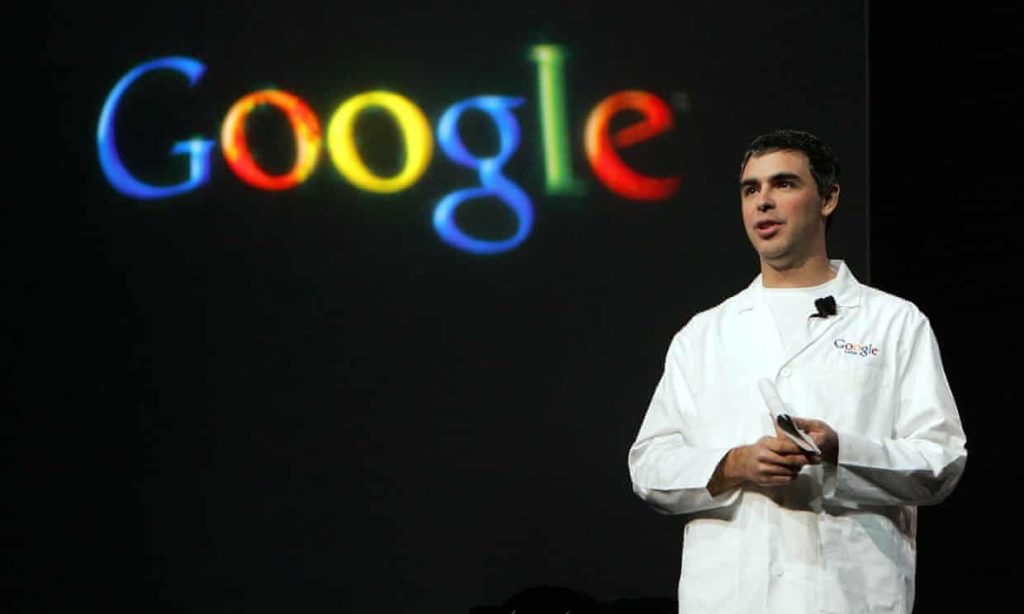
By David Sumpter is professor of applied mathematics at the University of Uppsala, Sweden, and author of The Ten Equations that Rule the World: And How You Can Use Them Too / theguardian.com